In the domain of tumor growth treatment, exact guess assumes a critical part in deciding patient results. Osteosarcoma, a threatening bone tumor growth, presents special difficulties because of its fluctuation in light of treatment.
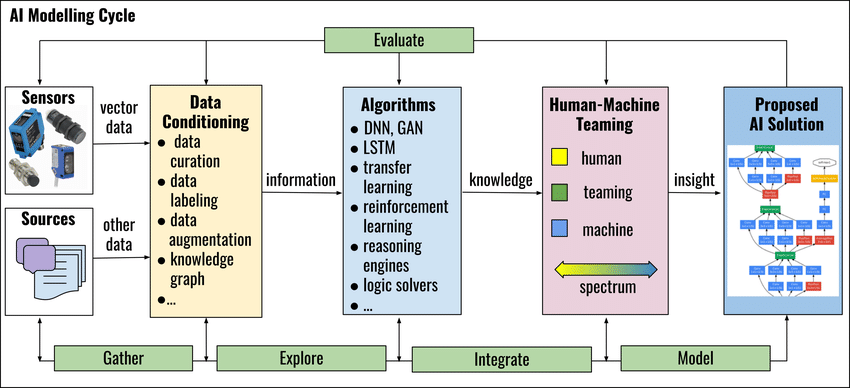
Customary strategies for surveying patient visualisation, for example, rot rate assessment by pathologists, frequently need accuracy and reproducibility. Be that as it may, scientists at Kyushu College in Japan have disclosed a weighty arrangement – an artificial intelligence equipped for assessing the thickness of enduring growth cells with unrivalled exactness. This development not just vows to upset prognostic evaluations for osteosarcoma patients yet in addition opens new roads for customised treatment methodologies.
Improved Prognostic Accuracy:
The traditional way to deal with assessing osteosarcoma forecasts depends on visual evaluations of necrotic tissue inside growths. In any case, this technique experiences inborn subjectivity and needs granularity in recognizing living and dead tumour cells. Perceiving these restrictions, the specialists at Kyushu College set out on fostering an artificial intelligence model that could give a more nuanced evaluation in view of individual cell counts. Through thorough approval utilising patient information, they demonstrated that their man-made intelligence model beat master pathologists in distinguishing practical growth cells, making them ready for upgraded prognostic exactness.
Key Proportions of Osteosarcoma Survival:
Those with high-thickness growth cells showed more terrible forecasts contrasted with their low-thickness partners, featuring the prescient force of the artificial intelligence model. Significantly, this approach delivered the customary corruption rate evaluation outdated, stressing the prevalence of suitable tumor cell thickness in gauging patient results.
Suggestions for Treatment Strategies:
The ramifications of this artificial intelligence driven development reach out a long ways past prognostic evaluations. By exactly breaking down growth pathology pictures, the model offers important experiences into the danger of osteosarcoma and individual tumor cell reactions to therapy. This, thus, empowers clinicians to artificial intelligence treatment techniques all the more really, possibly working on persistent results. Besides, the distinguishing proof of feasible tumor cells as a solid indicator of therapy reaction highlights the extraordinary effect of artificial intelligence in oncology.
Future Headings:
This addresses a huge step in the right direction in propelling the study of disease transmission, pathogenesis, and treatment methodologies for such malignancies. Eventually, the incorporation of artificial intelligence into oncology artificial intelligence is another period of accuracy medication, where prognostic evaluations are exact as well as custom-made to individual patient necessities.
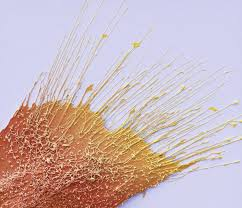
Credits: Inside Precision Medicine
- Training the Model:
- Split the data into training, validation, and test sets.
- Train the AI model on the training data, adjusting the model parameters iteratively to minimize the loss function.
- Validate the model performance on the validation set to avoid overfitting.
- Fine-tune the model as necessary based on validation results.
- Evaluation:
- Evaluate the trained model on the test set to assess its performance.
- Metrics for evaluation could include accuracy, precision, recall, F1 score, area under the receiver operating characteristic curve (AUC-ROC), etc.
- Additionally, consider domain-specific metrics or requirements relevant to tumor cell analysis.
- Iterative Improvement:
- Analyze the model’s performance and identify areas for improvement.
- Consider strategies such as data augmentation, model architecture changes, or hyperparameter tuning to enhance performance.
- Iterate through steps 3-5 until satisfactory performance is achieved.
- Deployment and Validation:
- Deploy the trained model in a real-world setting, ensuring that it integrates seamlessly with existing systems or workflows.
- Continuously monitor the model’s performance and gather feedback from users to identify any issues or areas for further improvement.
- Validate the model’s performance in the deployed environment to ensure consistency and reliability.
- Ethical and Regulatory Considerations:
- Ensure compliance with relevant regulations and ethical guidelines governing the use of AI in healthcare and medical research.
- Address concerns related to data privacy, bias, interpretability, and fairness in model development and deployment.
In the battle of artificial intelligence osteosarcoma, accuracy is principal. The coming of an artificial intelligence model equipped for assessing enduring tumor cells addresses a game-changing progression in prognostic evaluations.
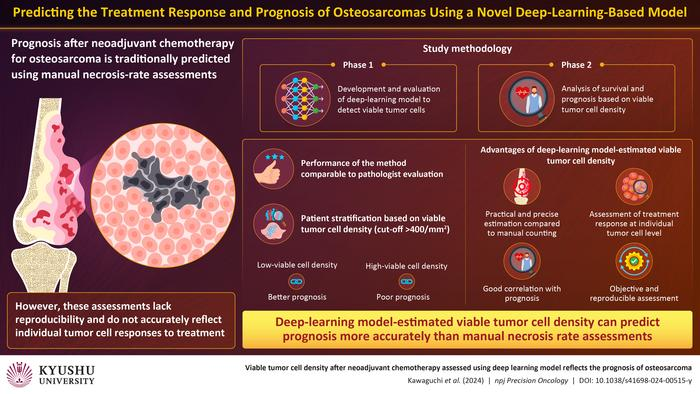
By rising above the impediments of conventional techniques, this advancement holds the possibility to rethink treatment procedures and work on persistent results. As specialists keep on unwinding the secrets of disease with the guide of man-made intelligence, the fate of oncology looks more splendid than any time in recent memory.
1 Comment
I don’t know where you get your knowledge, but this is a really great problem. I ought to take some time to comprehend or discover more. I appreciate the wonderful information; it was exactly what I needed for my purpose.